A data-driven framework to inform projections of aquatic flows in the Northeast
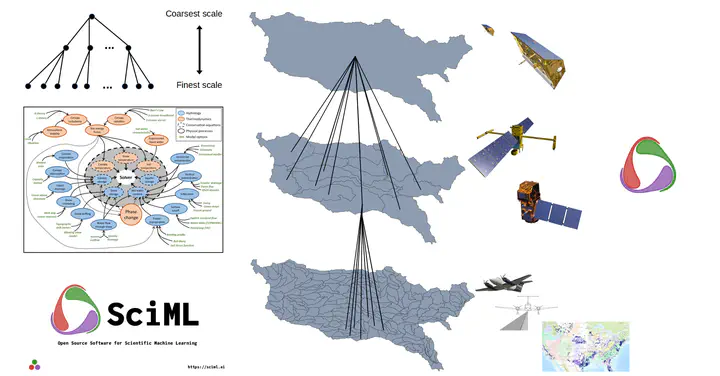
As climate change is impacting the future of aquatic flows, there is a great need for natural resource managers to assess adaptation measures in a holistic manner. The latter requires integrating multiple models with observational data, but that can be problematic due to disparities in terms of scale or fidelity of each model. Scientific machine learning, which has exploded in popularity in recent years, could provide a novel and flexible way of fusing observations and models. This project aims to develop a data-driven modeling framework that can help stakeholders incorporate the impacts of climate change into future predictions of aquatic flows. The proposed team will convene a working group composed of local, state and federal resource managers who are responsible for mitigating the impacts of climate change caused by extreme hydrologic events on fish and wildlife. Informed by this working group, the researchers will develop the data-driven framework by adopting modern computational methods that facilitate communication and ensure that the actual users of this framework are involved with its development from the ground up. The focus area of the proposed research will be the Northeast US, although the modeling tool is robust and can be applied within any region of the United States. Detailed user documentation and a simple interface will allow resource managers to incorporate the modeling tool into their existing workflow, making its adoption more likely. Data volumes are not getting any smaller, with more observations and model output becoming available to everyone. The overarching goal of this research is to bring in the very promising area of scientific maching learning into the hands of interested users and resource managers, ensuring fair access to such tools and demonstrating a different paradigm for climate change science.